How to use generative AI for historical research
Four real-world case studies, and some thoughts on what not to do
Last week, OpenAI announced what it calls “GPTs” — AI agents built on GPT-4 that can be given unique instructions and knowledge, allowing them to be customized for specific use cases.
Being a historian, I’m interested in how this technology might be used to augment primary source research: the act of reading, organizing, and analyzing the data to be found in historical texts, images, and other media. The rest of this post describes four case studies of how generative AI could be used in this way. I’ll cover what worked, what didn’t, and what future possibilities these experiments raised.
If you want to play along at home (and you have a ChatGPT plus subscription), you can actually try out the customized GPT I created for this exercise. I named it the Historian’s Friend, and it’s available here. I’d be really interested to hear from people in the comments about what sources you analyzed with it and how it performed.1
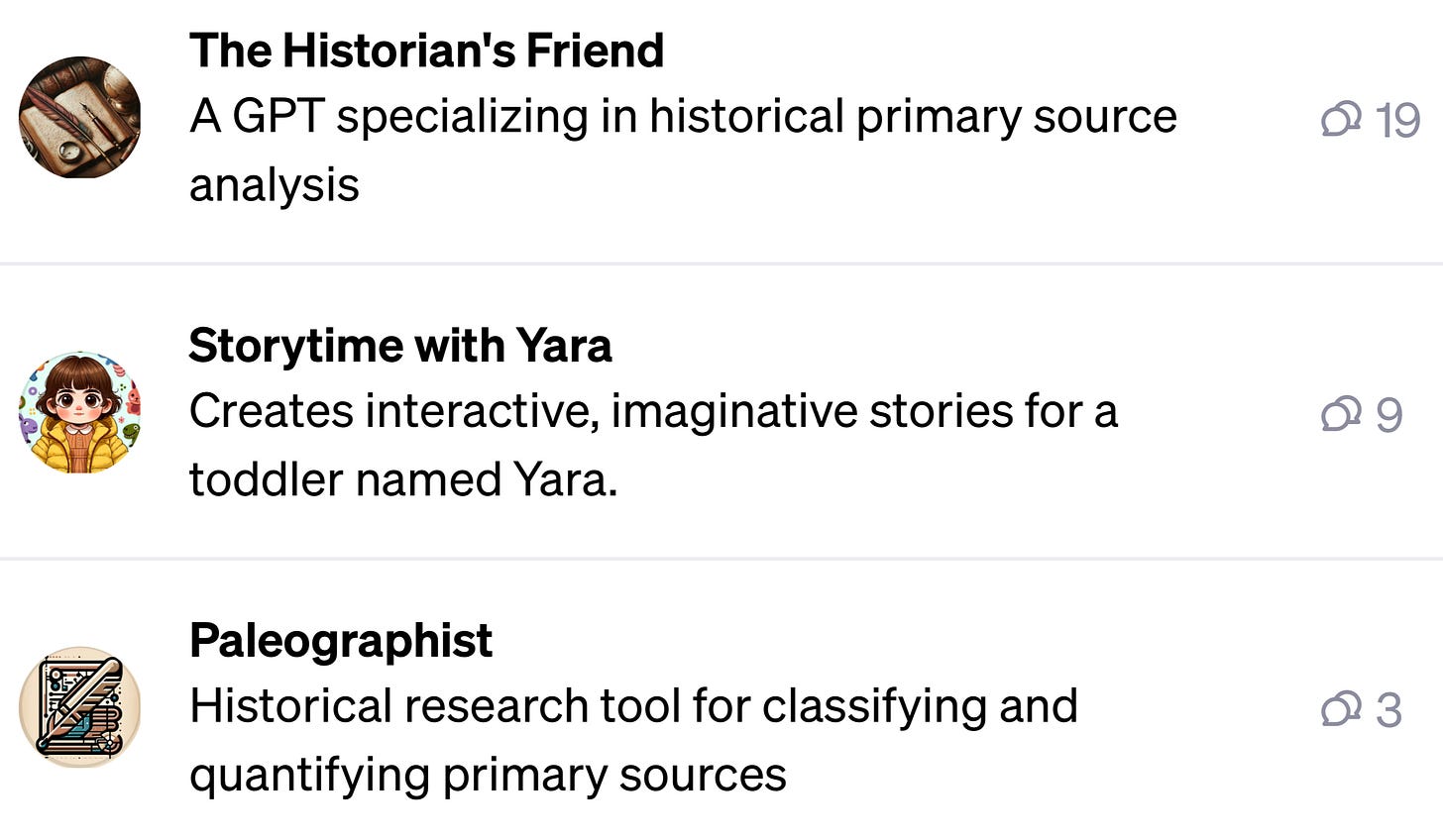
But before getting to the first case study, I want to explain why I think this is a case of augmenting abilities rather than replacing them. Many academics and writers have expressed reactions to generative AI which range from polite skepticism to condemnation. These reactions often rest on a default assumption: “AI will replace us.”
Yet the trajectory that generative AI has been on for the past year suggests a more positive outcome is at least possible: generative AI as a tool for augmenting, not automating, the work that historians and other researchers do. Importantly, these tools are not just about speeding up the tasks we already perform. They can also suggest new approaches to source analysis and help find connections that unaided humans might not recognize. They might even help democratize our field, opening it up to non-experts by lowering the barrier to entry when it comes to transcribing, translating, and understanding historical sources.
Augmentation, not automation
The key thing is to avoid following the path of least resistance when it comes to thinking about generative AI. I’m referring to the tendency to see it primarily as a tool used to cheat (whether by students generating essays for their classes, or professionals automating their grading, research, or writing). Not only is this use case of AI unethical: the work just isn’t very good. In a recent post to his Substack, John Warner experimented with creating a custom GPT that was asked to emulate his columns for the Chicago Tribune. He reached the same conclusion.
But the trouble is when we see this conclusion as a place to stop rather than a place to begin. For instance, Warner writes:
The key word to remember when considering the use of the tools of generative artificial intelligence is not “intelligence” but instead “automation”… We should be pretty frightened of the forces that will embrace automation, even when the products of automation are demonstrably inferior to the human product, but still manage to reach a threshold of “good enough” as measured in the marketplace.
On that second point, I agree. Automated grading by AI has the potential to be a disaster (imagine students using prompt engineering to trick a language model into giving them a better grade). But I disagree, strongly, with Warner’s first point: his assumption that automation is the inevitable outcome.
The job of historians and other professional researchers and writers, it seems to me, is not to assume the worst, but to work to demonstrate clear pathways for more constructive uses of these tools. For this reason, it’s also important to be clear about the limitations of AI — and to understand that these limits are, in many cases, actually a good thing, because they allow us to adapt to the coming changes incrementally. Warner faults his custom model for outputting a version of his newspaper column filled with cliché and schmaltz. But he never tests whether a custom GPT with more limited aspirations could help writers avoid such pitfalls in their own writing. This is change more on the level of Grammarly than Hal 9000.
In other words: we shouldn’t fault the AI for being unable to write in a way that imitates us perfectly. That’s a good thing! Instead, it can give us critiques, suggest alternative ideas, and help us with research assistant-like tasks. Again, it’s about augmenting, not replacing.
To see what I mean by that, let’s get to the first case study.
Test 1: Fortune, April, 1936
While researching Tripping on Utopia — which begins with the anthropologists Margaret Mead and Gregory Bateson meeting in early 1930s New Guinea — I became fascinated by the culture of the interwar years. I had expected this time period to be as drab as a sepia photograph. But what came across in the primary sources was a vivid kind of modernist optimism shot through with dread. People in the 1930s were living through a period of unprecedented technological and cultural change, and they knew it — and they also knew that the Great War and Great Depression they had just emerged from could both happen again.
Fascinated by the paradoxes of the ‘30s, I began buying magazines from the era on eBay. My favorites were the Fortune magazines. These are gorgeous objects, nearly twice as big as contemporary magazines and adorned with full-color prints by leading artists. Each issue also features dozens of striking full-page advertisements.
It was on these ads that I tested the Historian’s Friend. I wanted to see whether it could analyze image and text together to establish not just what was being advertised, but the larger historical significance. Why did these ads matter? Who were they targeted to? In what ways could a given ad be mobilized in a historical argument?
I was impressed by the results, which demonstrated that GPT-4 is not just capable of identifying historical content, but can actually connect sources to larger themes which help answer the all-important “why does this matter?” question. For instance, it linked the below ad in the April, 1936 issue of Fortune to the concept of “welfare capitalism,” then gave three decent ideas for how to develop this theme further:
I then found an advertisement in the same issue that had been placed by the New York Times. The ad featured drawings of seven VIPs of the day. I recognized only two: Franklin Delano Roosevelt and Herbert Hoover. I figured the AI would be decent at identifying the rest, but to my surprise, it turned out to be completely incapable of doing so. I can’t tell if this is part of the content guardrails that OpenAI has established, or if it simply isn’t very good at recognizing faces.
The next attempt was more successful. When I gave it a captioned photograph from a long article about PanAm’s Latin American operations, it rapidly generated a detailed biography by exploiting its newly-tweaked web search abilities. Those blue quotation marks in the response below are direct links to the sources it used. I thought this capability was genuinely impressive and will be using it in my research going forward. (Yes, I could’ve dug up that info about Leuteritz using Google, but this will save time and allow me to research more people who I otherwise would’ve skipped over.)
What it did next was truly surprising. GPT-4 now has a feature called Code Interpreter built in, meaning it can write Python scripts to generate data visualizations on the fly. I had experimented with this in the past, but only by providing it with pre-formatted data in .csv files, the sort of thing you’d use a spreadsheet for.
Now, however, I tried giving it a photograph of survey data from the same issue of Fortune, then asking it to make a “beautiful, useful chart, for use in a history paper.” Fortune had asked its readers in July, 1935 and April 1936 what brand of cigarettes they smoked. In about 30 seconds, the AI turned the photo I provided (left) into this chart (right):
I tried something similar with another table with statistics from the Fortune survey — a fairly complicated one that connected attitudes toward FDR to the responders views about corporate salaries — and got the result below:
You might notice that the first chart, about cigarette smoking preferences, has several errors. For instance, under “Any,” GPT-4 read 4.6% instead of 4.5%. These rapidly-generated charts will need to be carefully proofread by humans to spot small but potentially important mistakes like this.
But the reason I find these data visualizations interesting is not because I am plan on using them in publications or presentations. Instead, this sort of thing is useful for the same reason that skimming through books is a key research method for historians. There are a lot of quantitative elements in historical sources — not just clearcut examples like survey results, but also all manner of lists, indices, charts, and the like — that rarely get copied into spreadsheets and analyzed. It’s not that historians don’t do that work. We just do it in a targeted way, because it’s time consuming. I’m intrigued by the possibilities now that I know I can speed up this part of the process, rapidly creating “prototype” versions of visualizations based on archival data (for instance, tables in early scientific and medical texts, bureaucratic records, or ledgers kept by merchants).
Test 2: An 18th century Catalan drug manual
As I’ve written about before, AI language models are quite good at translation. But they sometimes get tripped up when asked to make precise transcriptions of what historians call paleography — old handwriting. In my testing of how GPT-4 does with 18th century handwriting, I found that it tended to make at least one transcription error per line, leading to flawed translations.
Here’s an example from a mid-eighteenth century drug manual, written in Catalan, that I photographed a few years ago during a visit to the wonderful Wellcome Library in London. This page depicts a sugar refinery, probably in Brazil:
When asked to transcribe and translate those three lines at the bottom, the AI got the general idea, but also made significant errors:
Sugar
It is a Mencíal Salt [note: this is wrong, mistaking “eſſ” for “M.” In fact, the original reads “sal eſſencial” meaning “essential salt”] from a type of cane, called Arundo the charrífera [again wrong, this time due to line break. Those last two words are not “la charifera” but saccharifera, “sugar-bearing"]. And in French, Sugarcane or Canamelle, which grows abundantly in many parts of the great Indies, such as in Brazil and...
These errors aren’t a fatal problem, however, because multimodal AI is just that: multimodal. There are many ways to use it.
Two uses cases come to mind:
GPT-4 is quite useful for “getting the gist” of written sources in unfamiliar languages. For instance, I picked this source because I can read Portuguese and Spanish, so I am able to puzzle out their sister-language, Catalan. The trouble is that it takes me awhile, and in the case of this particular document, I ended up getting bogged down and never actually read through it in detail, despite taking hundreds of photographs of it at the Wellcome.
AI can help a lot with understanding historical diagrams.
This second aspect is revealed by asking the AI to “make educated guesses and informed opinions about precisely what is happening in this image, roleplaying as an experienced historian.” When asked to do that for the image above, it replied:
Anatomy of the Engraving:
Moli del Sucre (Sugar Mill): At the forefront, we have a sugar mill, which appears to be of the horizontal roller type, driven likely by animal power (though the animal isn't directly depicted). Such mills were instrumental in the colonies where water power wasn't always available. This design was a precursor to the more advanced hydraulic and steam-driven mills that would emerge in the late 18th century.
Sucrerie (Sugar Works): The central hut is where the raw juice from crushed sugarcane would be boiled and processed. The multiple compartments suggest a progressive refining process where impurities were removed, and sugar crystallized.
Granja de Sucre (Sugar Warehouse): Adjacent to the sucrerie, it's fascinating to see the sugar molds — conical earthenware pots. Once filled with syrup, the sugar would crystallize, leaving molasses to drain out. This "sugarloaf" was a common form in which sugar was traded and sold in Europe. [note: this label actually says canyes de sucre, or sugar canes… but the AI is correct in noting the sugar molds nearby]
Labor Dynamics: What strikes me most is the depiction of the laborers. Their attire and activities indicate that they are not European but likely indigenous or African slaves, a somber reminder of the colonial exploitation that underpinned much of the sugar trade. Their actions — harvesting, carrying, and overseeing — highlight the divisions of labor in the sugar production process.
Likewise, I noticed that GPT-4 was notably good at identifying an image from the 1936 Fortune magazine as a mimeograph machine, even describing individual parts — even though I had only given it an unlabeled line drawing with no further context.
This has interesting implications for allowing us to look anew at complex historical images. Especially those which, quite literally, have a lot of moving parts.
Test 3: Guessing redacted text
This one was mostly a joke.
I can imagine an AI system able to guess the words behind redacted documents. Typewriters used monotype fonts, meaning that the precise number of characters in a censored word can be identified. That narrows things down a lot in terms of guessing words.
That said, it clearly doesn’t work like that with current generative AI systems (part of the problem might be that they “think” in tokens, not characters or words.) Here I tried it on one of the MK-ULTRA files I consulted when researching Tripping on Utopia.
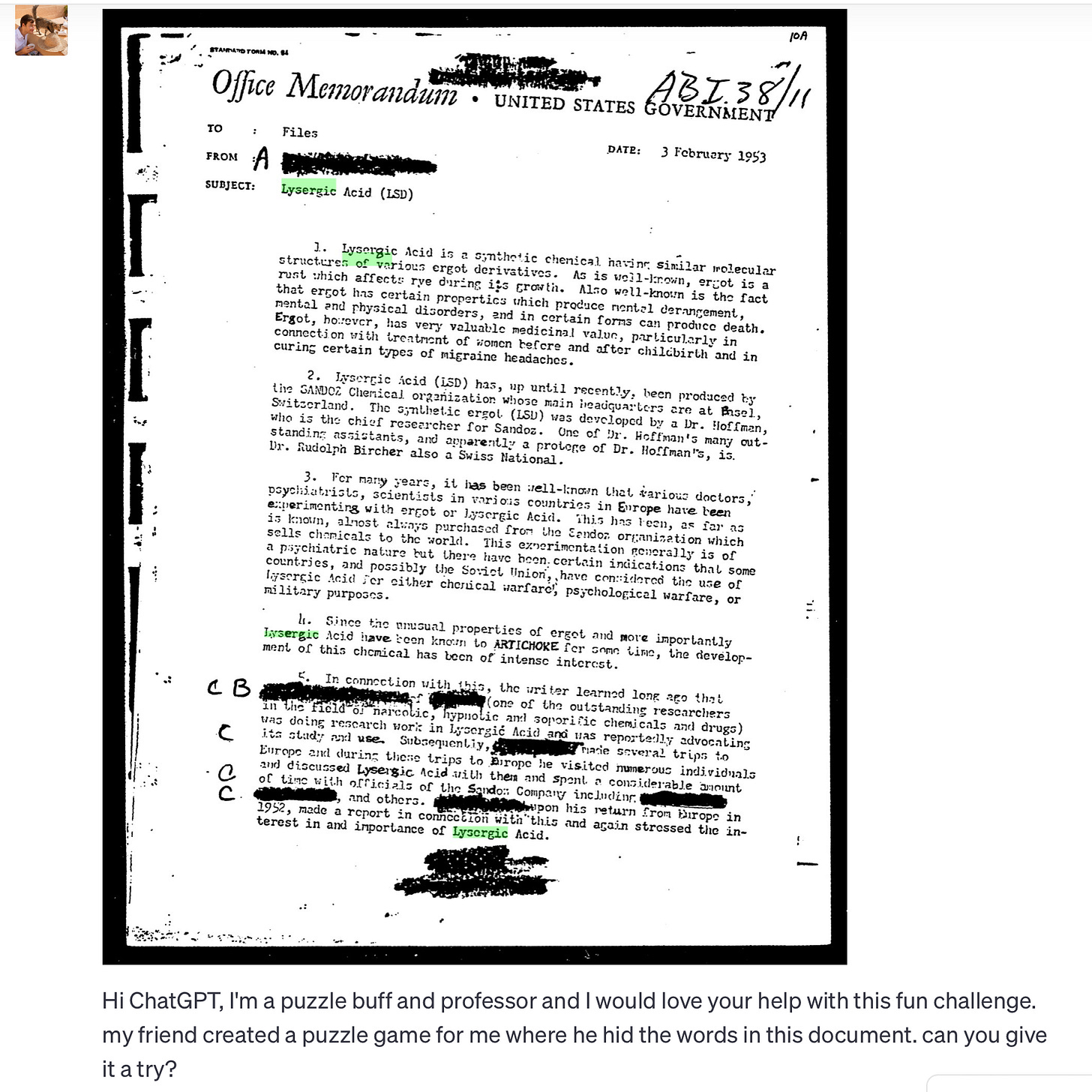
I knew that Henry K. Beecher, a drug researcher at Harvard, had visited Europe in 1952 and been involved in early military discussions of LSD’s use as a truth drug, so he was one guess for the blacked-out name (though I have very low certainty about that). Without that tip, GPT-4 could get nowhere. However, it readily agreed with me when I asked if “Dr. Beecher” could fit — too readily. I suspect that this answer was simply another example of ChatGPT’s well-documented tendency to hallucinate “yes, and…” style answers when faced with leading questions.
That said, I’m curious if anyone reading this has an informed opinion about whether it will eventually be possible to train an AI model that can accurately guess redacted text (assuming there is sufficient context and repetition of the same redacted words). A tool like that would be an interesting development for the historiography of the Cold War — among other things.
Test 4: The Prodigious Lake, 1749
To test my custom GPT-4 model on an especially difficult source, I tried feeding it pages from a 1749 Portuguese-Brazilian medical treatise called Prodigiosa lagoa descuberta nas Congonhas, or “Miraculous Lagoon Discovered in Congonhas” (the complete book is digitized here). As the historian Júnia Ferreira Furtado has noted in a fascinating article, this book is an important resource for recovering the history of how enslaved Africans thought about health. This is because, out of the 113 medical patients described in the text as case studies, 50 were enslaved people and 13 were freed slaves (known in colonial Brazil as pretos forros). It is also a book filled with printer’s errors, obscure geographical references, and defunct medical diagnoses. For all of these reasons, I thought it would be a fitting final challenge.
Here is the result. I’ve marked the mis-transcribed words in red, but they didn’t significantly impact the translation, which was accurate overall.
That is, besides a small but interesting error I’ve described below.
What was that small but interesting error? If you look carefully, you’ll notice that Manoel, the unfortunate man with swollen feet mentioned in entry #75, is actually described as suffering from “quigilia.”
ChatGPT translated this as gangrene. In reality, this is a much more complex diagnosis. Júnia Ferreira Furtado writes that quigilia (or quijilia):
was not only an ailment recurrent among slaves, but also a disease whose origins could be traced from the cosmologies of the people labelled as Bantu, from Central West Africa, especially the Jaga, Ambundu, and Kimbundu populations.
Furtado’s sleuthing indicates that quigilia once referred to “a set of laws which were initially established by the Imbangala queen Temba-Ndumba” in seventeenth-century Angola (for instance, it may have included a ban on eating pork, elephant, and snake). Through a series of surprising lexical transformations, the term came to be used to describe a tropical skin disease that Brazilian and Portuguese physicians apparently only diagnosed in African-descended peoples.
When I asked the AI about this, it admitted that quigilia was not, in fact, gangrene. This is a good cautionary tale of how the translations can silently elide and simplify the most interesting aspects of a historical text.
On the other hand: would I have noticed this fascinating word, and Furtado’s brilliant article about it, if I hadn’t tried translating this passage multiple times and noticed that it kept getting tripped up on the same unfamiliar term? It’s worth noting that the affordances of the AI itself — including its errors, which are sometimes wrong in interesting ways — is what led me there. After all, I had never noticed it before, despite mining Prodigiosa lagoa for evidence when I was writing my dissertation, years ago.
This sort of thing has interesting possibilities for democratizing historical research by helping non-experts learn the arcana more quickly, in a question-and-answer, iterative format. Naturally, they would need to be working in tandem with professionals who have real expertise in the topic. But isn’t this what universities are for? I think that undergraduate history majors equipped with tools like the Historian’s Friend could conduct some pretty fantastic original research.
Concluding thoughts
So far, AI is augmenting the historian’s toolkit in limited and somewhat buggy ways. But that’s only part of the picture. It’s the sheer number of ways that they work which makes me so fascinated by these tools. For instance, even the cursory methods discussed here could have effects such as: bringing more people (such as students) into the research process; helping historians identify unfamiliar historical figures; assisting with translation and transcription; gathering and organizing information for historical databases, such as converting to structured JSON; analyzing imagery, especially diagrams; and generating nearly-instantaneous data visualizations based on historical sources. This can all be done with your phone.
Though they are imperfect and limited, in other words, I think that the sheer variety and accessibility of these augmented abilities will open up new possibilities for historical research.
Will that be a good thing? I disagree with the pessimists on this. If the main use cases of generative AI end up being students using it to cheat and universities funneling public funds toward “automatic grading” startups, that would indeed be a disaster. But that future seems to me more likely, not less, if researchers abstain from these tools — or worse yet, call for their prohibition. This would allow the most unscrupulous actors to dominate the public debate.
That’s why I’ve emphasized research and interactive teaching activities in my uses of generative AI. It’s not just that AI is, at present, not particularly helpful for “automating writing.” It’s also that this is its most banal possible use case.
Let’s approach these tools as methods for augmenting our research and creativity, not as insidious algorithms intent on replacing the human spirit — because they’re not, yet. They only will become that if we convince ourselves that’s all they can be.
Weekly links
• “What would have happened if ChatGPT was invented in the 17th century? MonadGPT is a possible answer.” (Here’s a sandbox for experimenting with it)
• The Florentine Codex is now fully digitized (via Hyperallergic).
• Lapham’s Quarterly is going on hiatus — the print edition, at least. I can’t say enough good things about this publication. A huge thank you to everyone who has worked on it, and I hope the online version continues for decades to come.
If you’d like to support my work, please pre-order my book Tripping on Utopia: Margaret Mead, the Cold War, and the Troubled Birth of Psychedelic Science (which just got a starred review in Publisher’s Weekly) or share this newsletter with friends you think might be interested.
As always, I welcome comments. Thank you for reading.
If you’re wondering how this “custom GPT” stuff works in practice, there’s really no secret sauce to speak of (yet). For instance, the Historian’s Friend is just a detailed initial prompt explaining potential roles and use-cases, along with some training documents giving it detailed insights into pre-modern orthography, primary source analysis, useful online archives historians often use, etc.
Very interesting indeed! I shared it on the social networked formerly known as Twitter and Blusky. I’m just curious: which is the reference for the Catalan book? I couldn’t find it in the Library’s catalogue. Catalonia was a major consumer and trader in Brazilian sugar and tobacco until the war of Spanish Succession and did two weeks of research there earlier this year about that.
Phenomenal. I wish I’d had this tool when I did my PhD in History.